Anyone who uses a smartphone or shops online has had their habits tracked, click by telling click. Big companies comb through that data to find patterns in human behavior and to understand, anticipate, and offer up goods and services we are most likely to purchase. Through predictive analytics, they identify trends and forecast our future choices.
This high-tech data crunch has become increasingly common in higher education, too. Colleges and universities are facing mounting pressure to raise completion rates and have embraced predictive analytics to identify which students are at risk of failing courses or dropping out. An estimated 1,400 institutions nationwide have invested in predictive analytics technology, with spending estimated in the hundreds of millions of dollars. Colleges and universities use these analyses to identify at-risk students who may benefit from additional support.
How accurate and stable are those predictions? In most cases, college researchers and administrators don’t know. Most machine-learning models used in higher education are proprietary and operated by private companies that provide little, if any, transparency about the underlying data structure or modeling they use. Different models could vary substantially in their accuracy, and the use of predictive analytics could lead institutions to intervene disproportionately with students from underrepresented backgrounds. It’s also not clear whether these expensive services and complex models do a better job of identifying at-risk students than simpler statistical techniques that take significantly less time and expertise to implement and that institutions therefore may be able to implement on their own.
We put six predictive models to the test to gain a fuller understanding of how they work and the tradeoffs between simpler versus more complex approaches. We also investigated different approaches to sample and variable construction to see how data selection and model selection work together. Our study uses detailed student data from the Virginia Community College System to investigate whether models accurately predict whether a student does or does not graduate with a college-level credential within six years of entering school. Using these same models, we also examine, for a given student, whether their predicted risk of dropping out is the same from one model to the next.
We find that complex machine-learning models aren’t necessarily better at predicting students’ future outcomes than simpler statistical techniques. The decisions analysts make about how they structure a data sample and which predictors they include are more critical to model performance. For instance, models perform better when we include predictors that measure students’ academic performance during a specific semester or term than when we include only cumulative measures of performance.
Perhaps most importantly, we find that the dropout risk predictions assigned to a given student are not stable across models. Where students fall in the distribution of predicted risk varies meaningfully from one model to the next. This volatility is particularly pronounced when we use more complex machine-learning models to generate predictions, as those approaches are more sensitive to which predictors are included in the models and which students and institutions are included in the sample. For example, among the students considered at high risk of dropping out based on predictions generated from a linear regression model, just 60 percent were also deemed high risk according to a popular machine-learning prediction algorithm called XGBoost.
Finally, we show that students from underrepresented groups, such as Black students, have a lower predicted probability of graduating than students from other groups. While this could potentially lead underrepresented students to receive additional support, the experience of being labeled “at risk” could exacerbate concerns these students may already have about their potential for success in college. Addressing this potential hazard is not as straightforward as just removing demographic predictors from predictive models, which we find has no effect on model performance. The most influential predictors of college completion, such as semester-level GPA and credits earned, are correlated with group membership, owing to longstanding inequities in the educational system.
Our findings raise important questions for institutions and policymakers about the value of investments in predictive analytics. Are institutions getting sufficient value from private analytics firms that market the sophisticated models? Even more fundamentally, since a primary goal of predictive analytics is to target individual students with interventions to keep them on track to completion, how reliable are these methods if a student’s predicted risk is sensitive to the particular model used? Colleges and universities should critically evaluate what they are getting for their investment in predictive analytics, which one estimate puts at $300,000 per institution per year, as well as the equity implications of labeling large proportions of underrepresented students as being “at risk.”
Who Goes on to Graduate?
The predictive analytics boom has coincided with growing pressure on colleges and universities to raise completion rates. About two thirds of U.S. states now use performance-based funding, which bases a school’s annual state aid amount on the outcomes of its students, not the size of its enrollment. Meanwhile, students are borrowing record amounts of money to fund their postsecondary education, and loan default rates are highest among students who drop out before finishing their degree.
Institutions have turned to predictive analytics to determine which students are most at risk of dropping out and to more efficiently steer advising and other interventions toward students identified as needing help. Such resources are relatively scarce after a decade-long decline in higher education funding—particularly at the non-elite, broad-access colleges and universities where most lower-income and underrepresented students enroll. If predictive analytics perform as intended, institutions can more effectively and efficiently target resources for the students who need them most.
For that to work, predictions must be accurate. We tested six models to see which do a better job of assessing student risk and which sorts of decisions we could make along the way to make models more or less accurate. These include three models that are commonly used by researchers due to their ease of implementation and interpretation: Ordinary Least Squares, Logistic Regression, and Cox Proportional Hazard Survival Analysis. We also tested three more complex and computationally demanding models: Random Forest and XGBoost, which both use decision-tree learning as the building block to predict outcomes, and Recurrent Neural Networks, which applies layers of intricate patterns overtop one another to model complex relationships between data inputs and outcomes.
We test these models using detailed data for 331,254 community college students in Virginia, all of whom initially enrolled between summer 2007 and summer 2012 as degree-seeking, non-dual-enrollment students. We focus on predicting “graduation,” which we define as the probability that a student completes any college-level credential within six years. Some 34 percent of students in our sample graduated within six years, either from a community college or a four-year school. This rich dataset includes hundreds of potential predictors, including student characteristics, academic history and performance, and financial aid information, among others.
We observe each student’s information for the entire six-year window after the term when they initially enroll. While in all of our models we use the full six years of data to construct the outcome measure, we test two different approaches to constructing model predictors.
Choosing the Student Sample. First, we construct a sample using all information from initial enrollment through one of two concluding events: either the term when the student first earned a college-level credential or the end of the six-year window, whichever comes first. As an alternative approach, we constructed a randomly truncated sample of students so the distribution of enrollment spells in the model-building sample matches the distribution for currently enrolled students.
Choosing Predictor Variables. Second, we investigate how using more and less complex predictors affects model performance. First, we test models that use simple data points like race and ethnicity, parental education, cumulative GPA, and the number of courses completed. Then, we use those same models but supplement the simple variables with more complex predictors, such as measures of students’ enrollment at institutions outside the Virginia community college system.
We then test how model performance is affected by the inclusion of predictors whose values vary over time. We include both simple term-specific predictors like GPA or credits attempted and separately test the inclusion of complex term-specific predictors, like how academically demanding students’ courses are in a given semester and the trajectory of students’ academic performance over time. Our overall aim is to compare how model accuracy varies based on our choices of sample and predictor construction and modeling method.
Our primary measure of model accuracy is the c-statistic, also known as concordance value. This “goodness of fit” measure determines whether a model is, in fact, predictive of the outcome of interest. In our study, the c-statistic assesses whether a randomly selected student who actually graduated has a higher predicted score than a randomly selected student who did not. A c-statistic of 0.5 indicates that the prediction is no better than random chance, while a value of 1.0 indicates that the model perfectly identifies students who will graduate. The higher the score, the better; often, a c-statistic value of 0.8 or above is used to identify a well-performing model.

Predictions versus Reality
Our analysis finds that it is possible to achieve strong model performance with a simple modeling approach, such as Ordinary Least Squares regression. However, doing so requires thoughtful approaches to sample and predictor construction. Alternatively, it is possible to achieve strong performance with basic predictors, but doing so requires more sophisticated modeling approaches.
Using the relatively simple Ordinary Least Squares model as a baseline, we look closely at the improved accuracy of predictions made using more or less complex sampling and data selection (see Figure 1). Applying Ordinary Least Squares to the entire sample results in a c-statistic value of 0.76. That grows to 0.81 when using the sample that is “truncated” to be more representative of currently enrolled students with respect to their time enrolled in college and 0.88 when also including more comprehensive predictors.
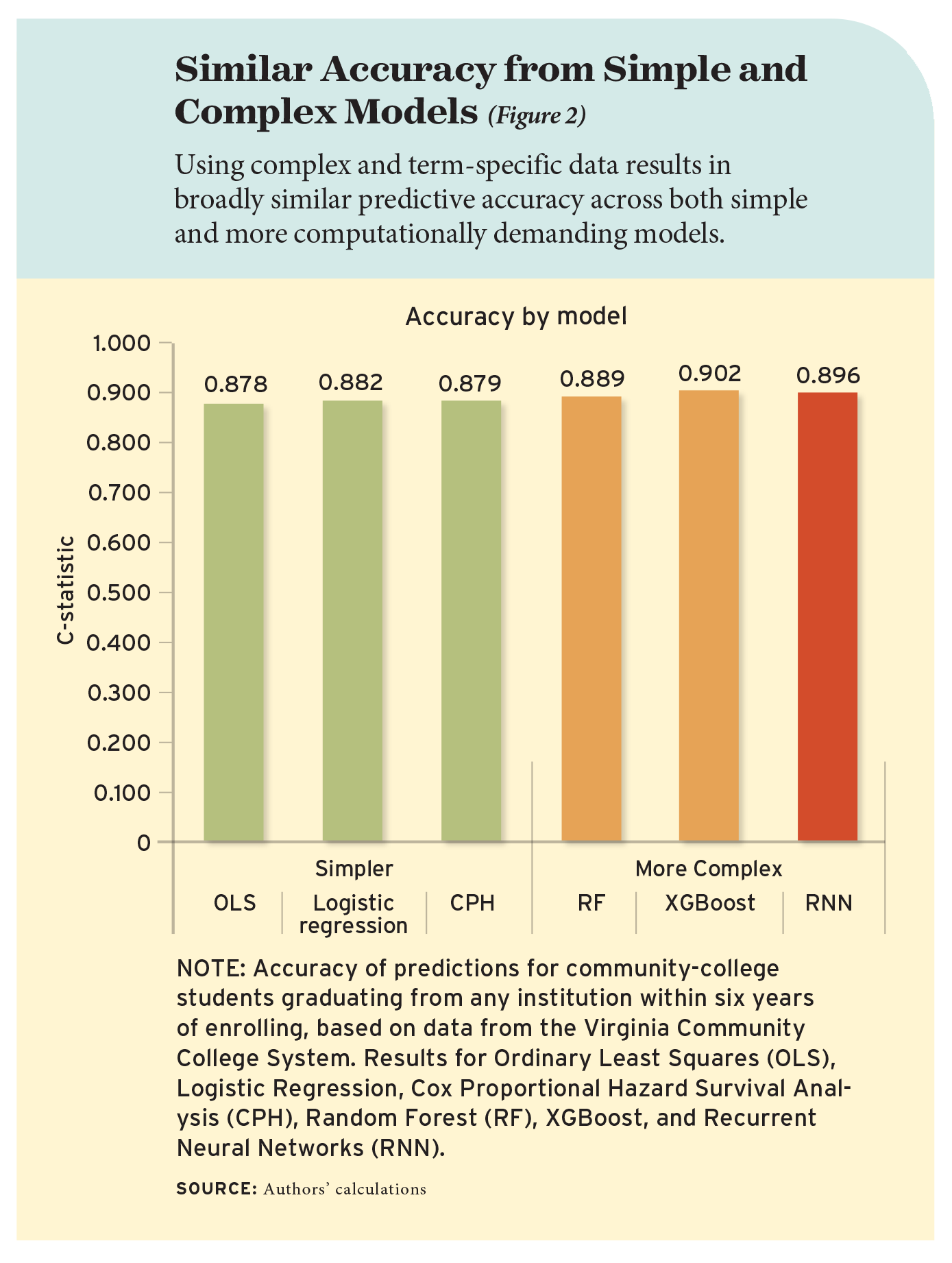
We apply the same truncated sample and set of comprehensive predictors to five additional modeling approaches to document the gains in accuracy from using more complex prediction algorithms (see Figure 2). The c-statistics are similar across the six models, ranging from 0.88 for the Ordinary Least Squares model to 0.90 for the more complex, tree-based XGBoost model. These fairly high values are not particularly surprising, given both the large sample size and detailed information we observe about students in the sample, but the fact that a basic model has nearly as high a score as a more complex model is notable.
To put this result in context, Figure 3 shows the number of students at a prototypical community college expected to be assigned a correct prediction across the different models we tested. Out of 33,000 students, Ordinary Least Squares would correctly predict the graduation outcomes of 27,119, or 82 percent. Three models perform a bit better: Logistic Regression, XGBoost, and Recurrent Neural Networks. XGBoost is the best-performing model and would correctly predict graduation outcomes for 681 more students than Ordinary Least Squares, a 2.1 percent gain in accuracy. The most computationally intensive model, Recurrent Neural Networks, presents the smallest gain over Ordinary Least Squares and would correctly predict outcomes for an additional 287 students.

A Question of Risk
One of the main purposes of predictive analytics is to identify at-risk students who may benefit from additional intervention. In predicting the likelihood of graduation for all students in our sample, each model also generates for each student a “risk ranking”—for example, that the student is at the 90th percentile among all students in terms of the probability of earning a degree. The higher the percentile value, the more likely a student is predicted to graduate relative to their peers. Students assigned lower predicted probabilities are therefore deemed at higher risk of dropout.
Colleges and universities may vary in which students they target for proactive outreach and intervention along the distribution of predicted risk. Some colleges may take the approach of targeting students at highest risk, while others may focus on students with more moderate predicted risk if they consider those students more responsive to intervention.
This raises a question about the relative accuracy of risk rankings. Regardless of where along the risk spectrum institutions choose to focus their attention, a desirable property is that different modeling strategies assign students similar risk rankings. How consistent are these rankings in practice from model to model?
We pair models together to compare where a student’s relative risk ranking falls. We divide the risk distribution into 10 equal groups, or deciles, and observe the extent to which students are assigned to different deciles across the two modeling approaches. For instance, among students whose predicted values from the Ordinary Least Squares model place them in the bottom 10 percent in terms of likelihood of graduation, we examine what percentage of those students are also assigned to the bottom 10 percent in the two other simple models. Some 86 percent of students in the bottom 10 percent based on Ordinary Least Squares are also in the bottom 10 percent from Logistic Regression. The same rate of consistency occurs between Logistic Regression and the third conventional model, Cox Proportional Hazard Survival Analysis.
However, discrepancies are more pronounced across all other model pairs. For example, half of students in the bottom 10 percent based on predictions from the tree-based Random Forest model are assigned to a different decile by the Recurrent Neural Network algorithm. We find even larger inconsistencies across models when considering students with lower predicted levels of risk. For example, across all model pairs, fewer than 70 percent of students assigned a risk rating in the third decile by one model were in that same decile by the other model.
If resource constraints prohibit colleges from intervening with all students predicted not to graduate, this instability in risk rankings means that the particular method of prediction used can significantly impact which students are targeted for additional outreach and support.
More Predicted Risk for Underrepresented Students
One common concern is that using predictive modeling in education may reinforce bias against subgroups with historically lower levels of academic achievement or attainment. In our sample, many historically disadvantaged groups—including Black and Hispanic students, Pell recipients, first-generation college goers, and older students—have significantly lower graduation rates than their more advantaged peers. At a conceptual level, including these types of demographic characteristics in predictive models could result in these subgroups being assigned a lower predicted probability of graduation, even when members of those groups are academically and otherwise identical to students from more privileged backgrounds.
This would likely result in students from disadvantaged groups being more likely to be identified as at-risk and provided additional supports. To be sure, if available interventions are effective, such identification could be a good thing. However, being flagged as “at risk” could be detrimental if it compromises students’ sense of belonging on campus, which is an important contributor to college persistence and success.
We examine how excluding demographic predictors affects model performance and student-specific risk rankings. It’s an intuitive approach to addressing this concern: without including demographics in predictive models, researchers and administrators might assume that students’ predicted outcomes would not vary by race, age, gender, or income. Furthermore, some state higher education systems and individual colleges and universities face legal obstacles or political opposition to including certain demographic characteristics in predictive models.
We compare the c-statistic values of models that include demographic characteristics to models that exclude this information and find their accuracy virtually unchanged. This occurs because many of the non-demographic predictors that remain in the model, such as cumulative GPA, are highly correlated with both student demographic characteristics and the probability of graduation. For example, Black students have a cumulative GPA of 2.13, on average, a half-grade lower than the 2.63 average of non-Black students. Even when race is not incorporated into prediction models explicitly, the results still reflect the factors that drive race-based differences in educational attainment. Institutions are therefore more likely to identify students of color as being at risk when using predictive analytics.
Questions to Consider
We believe there is a broad set of questions that are important for colleges and universities to consider when making decisions about using predictive analytics.
First, do the benefits of predictive modeling outweigh the costs? A back-of-the-envelope calculation can put this cost-benefit question in context. We find that using a more advanced prediction method like XGBoost would correctly identify graduation outcomes for an additional 681 students at a prototypical large community college that enrolls 33,000, compared to Ordinary Least Squares. If the cost to purchase proprietary predictive modeling services is estimated at $300,000, this implies an average cost per additional correctly identified at-risk student of $4,688. What other ways could institutions spend that money to boost completion rates? Are the potential benefits from sophisticated predictive analytics likely to be greater than those other investments?
Second, the instability in students’ relative risk ranking across models calls into question how strongly colleges should be relying on the “dropout risk” designation. In practical terms, this instability means that a student who is at substantial risk of dropping out may not get targeted for intervention, or a student who is predicted to have a higher probability of completion may get support they do not need. We encourage colleges and universities to advocate for greater transparency from their predictive analytics providers about the sensitivity of students’ relative risk rankings to different modeling choices. Choosing which prediction model to use may therefore depend, in part, on multiple factors, such as the intervention a college is developing, which set of students the college wants to target, and how closely the profile of students identified by a set of candidate prediction models comes to the target profile of students for intervention.
Third, students from underrepresented groups are likely to be ranked as less likely to graduate, regardless of whether demographic measures are included in the models. On the positive side, this could lead to institutions investing greater resources to improve outcomes for traditionally disadvantaged populations. But there is also the potential that outreach to underrepresented students could have unintended consequences, such as reinforcing anxieties students have about whether they belong at the institution. Colleges should weigh these considerations carefully.
Fourth, we see potential hazards regarding privacy and whether students are aware of and would consent to these uses of data. For instance, researchers at the University of Arizona constructed an experiment using machine learning to predict whether students dropped out before earning a degree with up to 90 percent accuracy based on their levels of campus engagement within the first few weeks of school. The source data: student ID swipes, which tracked their movements across campus—when they left their dorm rooms, checked out library books, or even bought a coffee. While this sort of data-gathering could have the potential to improve model accuracy, it also raises important privacy questions that higher education administrators need to actively consider.
A final question is whether predictive analytics is actually enabling more effective identification and support for at-risk students. Few studies to date have rigorously examined the effects of predictive analytics on college academic performance, persistence, and degree attainment; the few that do find limited evidence of positive effects.
However, it is easy to conflate the accuracy of predictive modeling with the efficacy of interventions built around its use. It could be that predictive models convey limited information about students, but it also may be the case that the resulting interventions were ineffective. While predictive analytics is intended to provide answers, we see further questions ahead.
Kelli A. Bird is research assistant professor at the University of Virginia, where Benjamin L. Castleman is Newton and Rita Meyers Associate Professor in the Economics of Education and Yifeng Song is data scientist. Zachary Mabel is associate policy research scientist at the College Board.